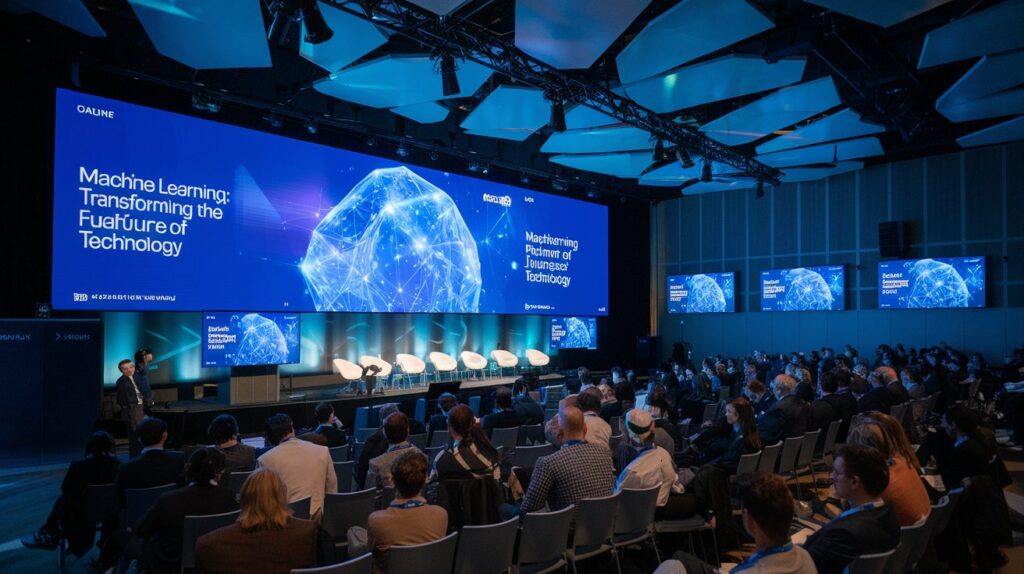
When a wider definition is applied, the subfield of artificial intelligence (AI), referred to as machine learning (ML), is emerging as a potent tool that allows systems to gradually refine outcomes based on data . It will be impossible not to emphasize that this ML Technology is the core underneath today’s innovations. ML is one of the most widespread technologies of the 21st century and it can help with recommendations, self-driving cars, and more. This article will introduce the reader into the basics of ML and its usages and prospects.
What is Machine Learning?
Machine learning can thus be defined as training the computer on how to discern characteristics contained in a given data set and make some conclusions based on such characteristics. Pivoting from traditional solutions, these ML Applications are now reshaping industries. Rather than simple instructions, it is informed by information in the field and adjusts its performance with operational experience.
There are three primary types of machine learning:
Supervised Learning: A process of training a given model on data that is already categorized with the known input and output. These include spam email detection as well as image recognition.
Unsupervised Learning: Works with unstructured data explaining the structure of images and text data. Some examples are; market segmentation analysis and fraud detection.
Reinforcement Learning: The main direction is to make decisions on trials and errors, it is used in robotics and games.
Machine Learning is applied in a number of ways, which include;
Healthcare: It is useful to make the right forecasting, diagnostics, treatment, and management of the processes occurring in healthcare organizations. ML is used in the diagnostics of diseases such as cancer, heart diseases. So, using predictive analytics as well as in drug development.
Finance: In the financial context, uses of ML include; fraud detection, algorithmic trading and credit scoring. Within a day, the large volume of the transaction data goes through ML models. That flag cases of fraud andput in place algorithms that check for patterns that look like fraud.
Retail and E-commerce:Recommendation engines that conduct targeted shopping. That is one of the most striking examples of the application of ML. And guess what, the platforms like Amazon & Netflix make use of ML for targeting the user behaviour and subsequently. The products or the content being recommended.
Autonomous Vehicles: Self-driving cars depend on machine learning to analyze the data collected from sensors. And to identify objects on the road, in addition to deciding when to steer, brake or accelerate. Today, leaders in the development of this technology include Tesla and Waymo firms.
Natural Language Processing (NLP): Machine learning underpins application such as voice recognition apps (e.g., Alexa, Siri); language translation; and sentiment analysis. NLP models allow machines to comprehend and analyze human language and therefore improve interaction.
Cybersecurity: Cybersecurity is improved by machine learning since the system is able to recognize risks and weaknesses within the computer networks. Anomaly detection models with the ability to discern, the problem activities stops data breaches and hacking.
This paper aims at describing the various challenges that are currently being experienced in the field of machine learning.
Data Quality and Quantity:
For training of ML models, large and clean data set is needed. This suggests that poor data quality means poorer performance, and less accurate prediction or forecasts.
Interpretability:
Most of the current ML models, especially deep learning algorithms work in a way that their decision-making mechanism is very much difficult to explain.
Ethical Concerns:
The following ones are ethically questionable: privacy, bias, and accountability. When it comes to the use of Machine Learning, this aspect is central in guaranteeing that this kind of process is fair and easily explainable.
A detailed look at the future of machine learning
Few emerging trends for machine learning are as follows: the use of quantum computing, federated learning, and automated machine learning (AutoML). These innovations seeks to improve the efficiency, scalability and usage of the ML models. This is because industries are gradually adopting machine learning and its ability to solve and contribute to complex challenges is bound to increase.
Conclusion
Machine learning is a revolutionary technology with pros in almost every industry. The future advancement in Machine Learning is already being described as one that would revolutionize industries and life. It is now touching on almost every aspect of life from healthcare to cybersecurity and altering industries and providing solutions for challenges. Firstly, various opportunities and concerns are painted, which show how this field may bring improved prospects and bridge the ethical and innovative gaps in our society.